Taking on the Data Challenge

Ingo Waldmann of UCL (UK) explains how launching a data challenge linked to the Ariel mission has led to new approaches and collaborations.
Read article in the fully formatted PDF of the Europlanet Magazine.
It is fair to say that machine learning, and in particular deep learning, has revolutionised data analysis in many fields of science and industry. Planetary science and exoplanet research are no exception; though our community may have been a little late in joining the party, the number of planetary science publications using machine learning is now increasing exponentially.
Papers on theoretical machine learning, potentially relevant to our field, are also being published at a similar rate. Keeping abreast of two rapidly moving subjects is nearly impossible and this is perhaps one of the main challenges faced today by interdisciplinary researchers.
Fortunately, we don’t have to be seasoned experts ourselves and can collaborate instead. However, finding the right person with the right data analysis expertise is reminiscent of searching for the proverbial needle in a haystack, particularly if you require a fresh perspective on long-standing issues and are not quite sure what you are looking for. From the machine learning community’s perspective, astronomy and planetary sciences can often be perceived as complex and challenging, so there is not much incentive to get involved.
As part of the team behind the European Space Agency’s Ariel mission, we have grappled with these issues for a while and, in 2019, we had an idea. What if we could package an unresolved problem into a data challenge aimed at a machine learning audience? By focusing on a smaller, bite-sized aspect of a larger problem, we could make the general subject of exoplanets more accessible. The data challenge is not designed to solve our data analysis issues outright, but to provide a forum that encourages future collaborations and hopefully bring new perspectives to the table.
Ariel will observe the atmospheres of approximately 1000 planets around stars other than our Sun. The mission aims to determine how exoplanets form and evolve, and put our own Solar System into context. Detecting starlight filtered through the atmosphere of a planet that may be hundreds of lightyears away is a challenge, particularly when signals from the star and the spacecraft itself can cause distortions.
In our first challenge, we chose to focus on the issue of separating out the stellar signal from the detections of the exoplanet’s atmosphere. Although some analytical approaches exist, it remains an inherently complex problem due to the ever-changing nature of the stellar and planetary signals under scrutiny. Instead of jumping in at the deep end with real-world observations, we built a more sanitised version first: a scalable simulation that captures some of the main issues of disentangling the data. We ran our simple star-planet simulation through the ESA Ariel mission simulator, built a competition website, and submitted a proposal to Europe’s leading machine learning conference: the somewhat clunkily named European Conference on Machine Learning and Principles and Practice of Knowledge Discovery in Databases (ECML-PKDD). Much to our delight, we were selected as one of their official ‘Discovery Challenges’.
So, what lessons have we learned from running a data challenge? Well, first, we were very surprised by the interest in taking part. In the first year, we had over 100 teams participating globally. When we repeated the challenge in 2021, with a more complex simulation, we hit 130 teams. We became one of the biggest challenges of the conference in recent years. It seems that people like studying planets (but perhaps offering a cash prize also helped a little). Another pleasant surprise was the significant media interest the challenge enjoyed. The name of the 2021 winner, Luís Simões (running his own AI company, ML Analytics) was featured in the Portuguese quiz show ‘Joker’ as the answer to the final question. The contestant won the jackpot as he had read about the challenge in the news.
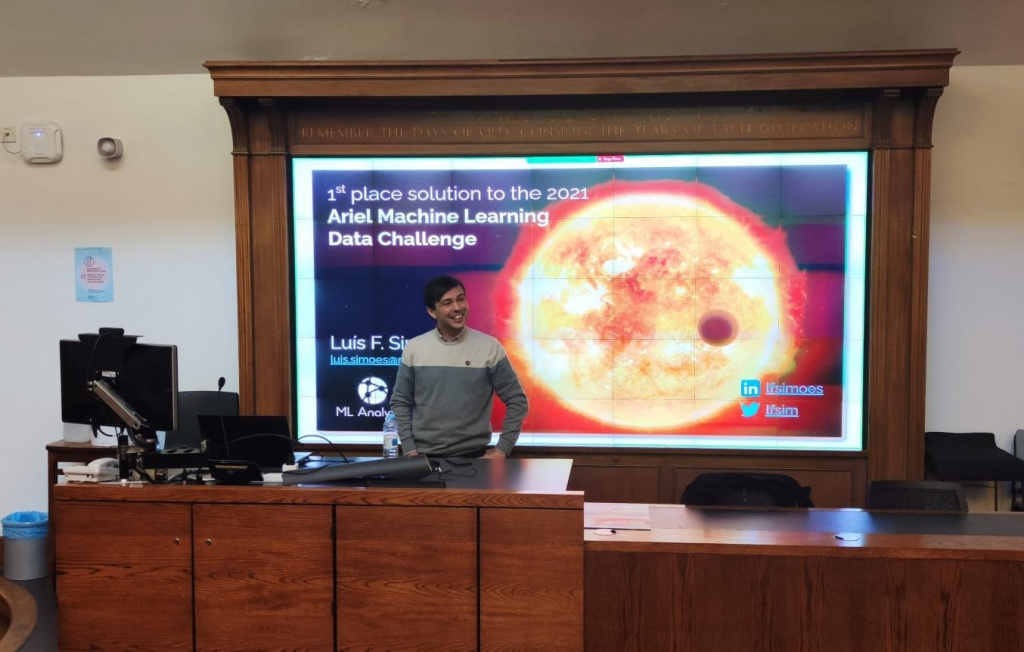
Scientifically it resulted in what we hoped for – new collaborations and some novel approaches to long-standing issues. The results were presented at two dedicated workshops at ECML-PKDD and the Europlanet Science Congress (EPSC), and also published in peer-reviewed journals. Of course, there’s no such thing as a free ride. Going into this, somewhat naïvely, we quickly realised that designing and running the challenge is equivalent in workload to organising a small conference. The need for careful planning was a lesson learned the hard way!
In spite of the time and effort to organise something on this scale, we have decided to run a data challenge or hackathon every year until the Ariel mission launches in 2029. It is surprisingly enjoyable watching the leaderboard evolve each day. In my opinion, it is one of the best ways to build interdisciplinary bridges and get the machine learning community excited about planetary sciences. This year we have proposed a challenge to the Neural Information Processing Systems (NeurIPS) conference 2022. The start date is the 15th of June. Hope to see you on the leaderboard!